My Jotwell Review of the ACUS Report Government by Algorithm
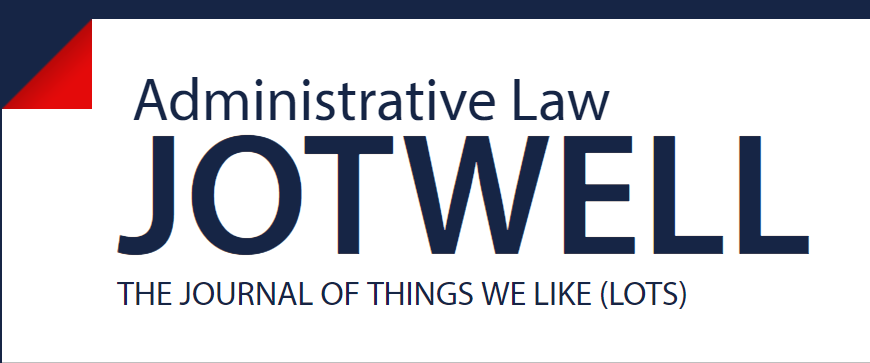
Over at the Journal of Things We Like (Lots) (Jowell), I have a short review of an important new report that the Administrative Conference of the United States (ACUS) has issued on artificial intelligence and machine learning in the federal administrative state. This report, entitled Government by Algorithm: Artificial Intelligence in Federal Administrative Agencies, was coauthored by David Freeman Engstrom, Daniel E. Ho, Catherine M. Sharkey, and Mariano-Florentino Cuéllar.
Here’s a taste of my review:
There is so much to like *lots* in this report—too much to cover in this short Jot. But I’ll flag a few highlights.
Part I of the report takes inventory of AI/ML across the federal bureaucracy. To do so, the researchers—including the report authors and a large team of law students, political scientists, and computer scientists—focus on the 142 most significant federal agencies. They find that 45% (64 agencies) have embraced AI/ML to some degree. As depicted in Figure 2 of the report, the most common use of AI/ML is for “regulatory resource, analysis, and monitoring,” followed by (in order) enforcement, public services and engagement, internal management, and adjudication. Roughly half of AI/ML use cases (84 of 157) were developed in house. Part I also breaks down the data by agency, subject matter, and implementation stage, among other things. A 43-page online appendix provides even more granularity, including details on all 157 use cases identified at these agencies. In reviewing the findings, I was struck by the fact that most agencies (though not all) experimenting with AI/ML have substantial resources. Many under-resourced agencies, such as agencies focused on immigration and veterans affairs, don’t seem to be doing much AI/ML innovation. I’ll return to this observation later.
***
A critical challenge to developing AI/ML internally, as I hinted above, is that federal agencies face significant budget constraints. Buying off-the-shelf solutions may thus be more tempting for agencies with limited resources, yet such outsourcing often carries greater risks from a Calo-Citron legitimacy perspective. And some agencies that may benefit the most from AI/ML innovations face greater budgetary challenges than others, such that buying AI/ML is not even possible. In the final implications category, the report wisely observes that make or buy are not the only options. Federal agencies can also borrow. These approaches include collaborating with outside non-commercial entities to develop AI/ML, sponsoring AI/ML innovation competitions, and collaborating with other administrative agencies. This borrowing category deserves much more attention in the literature and in the real world. Indeed, we may find similar parallels and helpful lessons from the history of military software procurement, as my colleague Bryan Choi explores in Software as a Profession, where the government pursued such a hybrid development and procurement strategy.
Government by Algorithm is a massive contribution to the administrative law literature, and it lays the foundation for an ambitious research agenda for this emerging subfield of administrative law. The report could not have come at a better time, as federal agencies turn more and more to AI/ML to regulate.
My full Jotwell review is here, and definitely go read the full report here.